标签:shape sdi namespace ids 图片 NPU clu with import
数据分析与建模,本次尝试使用C++进行处理,数据在excel中,遂考虑使用Python进行excel转txt操作,代码如下:
1 # -*- coding: UTF-8 -*-
2 import sys
3 import json
4
5 import pandas as pd
6 import numpy as np
7
8 filename=r‘C:\Users\lenovo\Desktop\that.txt‘
9 raw_score = r‘C:\Users\lenovo\Desktop\data1.xlsx‘
10 #读取excel保存成txt格式
11 excel_file = pd.read_excel(raw_score)
12 excel_file.to_csv(filename, sep=‘ ‘, index=False)
Python聚类分析代码:
1 import numpy as np
2 import matplotlib.pyplot as plt
3
4 # 加载数据
5 def loadDataSet(fileName):
6 data = np.loadtxt(fileName,delimiter=‘ ‘)
7 return data
8
9 # 欧氏距离计算
10 def distEclud(x,y):
11 return np.sqrt(np.sum((x-y)**2)) # 计算欧氏距离
12
13 # 为给定数据集构建一个包含K个随机质心的集合
14 def randCent(dataSet,k):
15 m,n = dataSet.shape
16 centroids = np.zeros((k,n))
17 for i in range(k):
18 index = int(np.random.uniform(0,m)) #
19 centroids[i,:] = dataSet[index,:]
20 return centroids
21
22 # k均值聚类
23 def KMeans(dataSet,k):
24
25 m = np.shape(dataSet)[0] #行的数目
26 # 第一列存样本属于哪一簇
27 # 第二列存样本的到簇的中心点的误差
28 clusterAssment = np.mat(np.zeros((m,2)))
29 clusterChange = True
30
31 # 第1步 初始化centroids
32 centroids = randCent(dataSet,k)
33 while clusterChange:
34 clusterChange = False
35
36 # 遍历所有的样本(行数)
37 for i in range(m):
38 minDist = 100000.0
39 minIndex = -1
40
41 # 遍历所有的质心
42 #第2步 找出最近的质心
43 for j in range(k):
44 # 计算该样本到质心的欧式距离
45 distance = distEclud(centroids[j,:],dataSet[i,:])
46 if distance minDist:
47 minDist = distance
48 minIndex = j
49 # 第 3 步:更新每一行样本所属的簇
50 if clusterAssment[i,0] != minIndex:
51 clusterChange = True
52 clusterAssment[i,:] = minIndex,minDist**2
53 #第 4 步:更新质心
54 for j in range(k):
55 pointsInCluster = dataSet[np.nonzero(clusterAssment[:,0].A == j)[0]] # 获取簇类所有的点
56 centroids[j,:] = np.mean(pointsInCluster,axis=0) # 对矩阵的行求均值
57
58 print("Congratulations,cluster complete!")
59 return centroids,clusterAssment
60
61 def showCluster(dataSet,k,centroids,clusterAssment):
62 m,n = dataSet.shape
63 if n != 2:
64 print("数据不是二维的")
65 return 1
66
67 mark = [‘or‘, ‘ob‘, ‘og‘, ‘ok‘, ‘^r‘, ‘+r‘, ‘sr‘, ‘dr‘, ‘‘, ‘pr‘]
68 if k > len(mark):
69 print("k值太大了")
70 return 1
71
72 # 绘制所有的样本
73 for i in range(m):
74 markIndex = int(clusterAssment[i,0])
75 plt.plot(dataSet[i,0],dataSet[i,1],mark[markIndex])
76 plt.annotate(i+1,(dataSet[i,0],dataSet[i,1]))
77
78 mark = [‘Dr‘, ‘Db‘, ‘Dg‘, ‘Dk‘, ‘^b‘, ‘+b‘, ‘sb‘, ‘db‘, ‘‘, ‘pb‘]
79 # 绘制质心
80 for i in range(k):
81 print(‘质心:‘,str(centroids[i,0])+‘ ‘+str(centroids[i,1]))
82 plt.plot(centroids[i,0],centroids[i,1],mark[i])
83 plt.title(‘Clustering map of specialists‘)
84 plt.show()
85
86
87 dataSet = loadDataSet(r‘C:\Users\lenovo\Desktop\normal.txt‘)
88 k = 3
89 centroids,clusterAssment = KMeans(dataSet,k)
90
91 showCluster(dataSet,k,centroids,clusterAssment)
聚类结果:
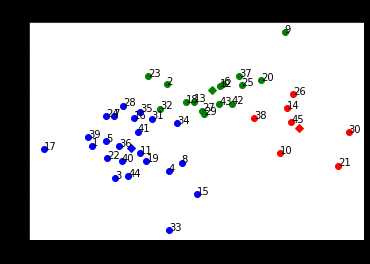
话说,c++建模也还可,就是需要自己编写相关指标的算法,但是也挺有意
代码如下:
1 /*
2 *language:c++
3 *version:11
4 *encoding:GBK
5 *made by Luo Wenshui
6 *last modified:2020/5/10
7 *data file:input.txt
8 */
9 #include 10 #include
使用Python将excel文件中的数据提取到txt中
标签:shape sdi namespace ids 图片 NPU clu with import
原文地址:https://www.cnblogs.com/randy-lo/p/12868259.html